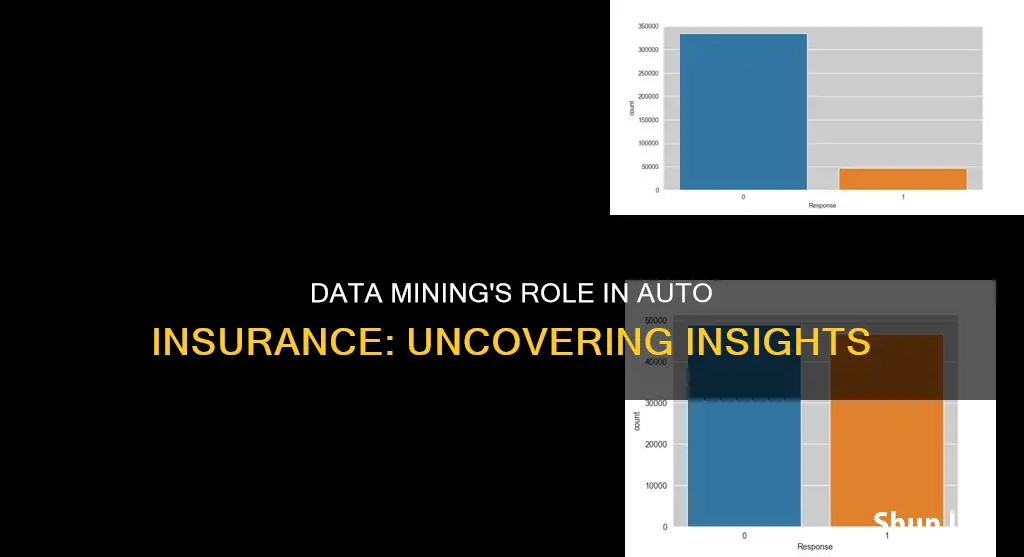
Auto insurance companies have been using data mining to set insurance rates and create risk-based insurance premiums. This involves collecting and analysing large amounts of data, including customer information, claims records, and vehicle characteristics. By employing machine learning and artificial intelligence techniques, insurance companies can identify patterns and make predictions about future claims. This helps them to assess risk, set premiums, and make data-driven business decisions.
One of the main objectives of data mining in auto insurance is to predict the likelihood and expected value of future claims for each insured individual. This is achieved by analysing various attributes, such as customer demographics, vehicle information, and claim history. Additionally, data mining can assist in fraud detection, customer acquisition, and pricing decisions.
While data mining offers significant advantages to insurance companies, there are also concerns about fairness and privacy. Critics argue that data mining can lead to unfair discrimination, with certain groups of customers being charged higher rates based on factors such as socioeconomic status or financial literacy.
Characteristics | Values |
---|---|
Data Collection | Unstructured data (PDFs, text documents, images, and videos) and structured data |
Data Management | Collection, cleansing, consolidation, and storage |
Data Analysis | Risk-based insurance premiums, fraud detection, customer acquisition, pricing and actuarial decisions |
Data Modelling | Survival analysis model, linear regression model, machine learning methods |
Data Sources | Internal data, external data from data aggregators, online data sources |
What You'll Learn
- Data mining is used to set insurance rates and premiums
- Data mining can be used to detect insurance fraud
- Data mining can help insurance companies identify trends and spot new opportunities
- Data mining can be used to improve customer service and reduce customer churn
- Data mining can be used to streamline the process of obtaining insurance quotes
Data mining is used to set insurance rates and premiums
Data mining is used by auto insurance companies to set insurance rates and premiums. This process is known as "price optimisation", and involves using sophisticated software to analyse large amounts of data to determine the likelihood and expected value of future claims for each insured individual. This data includes a myriad of attributes about the customers and their peers, such as demographic information, lifestyle characteristics, and risk information.
By employing data mining techniques, insurance companies can automate and improve data analysis, creating useful predictive models. These models can be used to identify new underwriting parameters and distinguish between different groups of policies. Ultimately, this allows insurance companies to set risk-based insurance premiums, with the most risky individuals being charged higher premiums than those deemed less risky.
For example, a study by Kahane et al. demonstrated that data mining techniques could be used to predict the average number of accidents and thefts per year for each insured individual. The results showed that the most risky individuals were more than 12 times more expensive to insure than the least risky individuals.
While insurance companies argue that price optimisation is a way to improve efficiency and precision in rate-setting, consumer advocates have criticised the practice as unfair and discriminatory. They argue that price optimisation allows insurers to charge higher rates to customers who are less likely to shop around for a new policy, including low-income individuals. As a result, there have been calls to ban the use of price optimisation in setting insurance rates and premiums.
Auto Insurance and Mileage: What's Deductible?
You may want to see also
Data mining can be used to detect insurance fraud
Data mining is a powerful tool that can be leveraged to combat insurance fraud, which is a significant issue within the insurance industry. Healthcare insurance fraud, for instance, results in billions of dollars in losses globally each year. By utilising data mining techniques, insurance companies can identify anomalies and patterns that indicate fraudulent activities. This involves analysing extensive data, including patient records, billing information, and medical claims, to uncover deceptive practices such as identity theft, billing for services not rendered, or collusion among healthcare providers.
Data mining techniques, such as association rule mining and unsupervised learning algorithms, play a crucial role in fraud detection. Association rule mining helps identify frequent patterns and relationships within the data, while unsupervised learning algorithms, like clustering and anomaly detection, can flag suspicious activities and outliers. These techniques enable insurance providers to make informed decisions and take appropriate actions against fraudulent claims.
The process of data mining for fraud detection typically involves several steps. Firstly, data is collected from various sources, such as patient records, insurance claims, and medical reports. This data is then preprocessed, which includes tasks such as data cleaning, normalisation, and feature selection. After preprocessing, data mining algorithms are applied to uncover hidden patterns and relationships. The detected anomalies or suspicious patterns are then investigated further to determine if fraudulent activities have occurred.
One example of data mining for insurance fraud detection is the use of machine learning algorithms to analyse insurance claims forms. These algorithms can identify changes or discrepancies in the claims forms, such as altered information or forged signatures. Additionally, natural language processing (NLP) techniques can be employed to analyse text-based data, such as adjuster notes or social media posts, to identify potential red flags or patterns indicative of fraud.
In conclusion, data mining is a valuable tool for insurance companies to detect and prevent fraud. By leveraging data mining techniques, insurance providers can protect themselves and their customers from financial losses due to fraudulent activities. However, it is important to continuously adapt and improve fraud detection methods as fraudsters also evolve their tactics over time.
Switching Auto Insurance While on Loan
You may want to see also
Data mining can help insurance companies identify trends and spot new opportunities
Firstly, data mining can be used to design risk-based insurance premiums for motor vehicles. By employing data mining methods, insurance companies can predict the likelihood and expected value of future claims for each insured individual based on a multitude of attributes available in the database on the customers and their peers. This information can then be used for underwriting and rate-making. For example, data mining can be used to estimate the probability of filing a claim and the average claim value, allowing insurance companies to distinguish between high-risk and low-risk customers.
Secondly, data mining can aid in customer acquisition and retention. By analysing customer characteristics and profiles, insurance companies can improve their marketing decisions and develop targeted offerings. Data mining can also help identify cross-selling and upselling opportunities, such as offering a life insurance policy to a customer with car insurance.
Thirdly, data mining can be used to detect fraud. Insurance companies can use data mining techniques to analyse insurance applications or claims forms for red flags and identify potential fraud cases.
Lastly, data mining can help streamline the process of claims processing. By automating the analysis of claims forms, data mining can reduce the time and costs associated with manual processing.
Overall, data mining provides insurance companies with valuable insights that can inform their business strategies and enable them to identify new opportunities for growth and improvement.
The Phoenix Conundrum: How the City's Rapid Growth Impacts Auto Insurance Rates
You may want to see also
Data mining can be used to improve customer service and reduce customer churn
Data mining can be used by auto insurance companies to improve customer service and reduce customer churn in several ways.
Firstly, data mining can help insurance companies identify customer trends and be better prepared to support their customers. By understanding customer preferences, behaviours, and patterns, insurance providers can fine-tune their products and services to meet and exceed customer expectations, thereby improving customer satisfaction and reducing churn.
Additionally, data mining can assist insurance companies in gauging customer likes and dislikes, allowing them to make informed decisions that increase brand loyalty. For example, by using data mining to determine which groups of customers are more likely to accept a price increase, insurance companies can avoid alienating customers with unnecessary rate hikes, thus reducing churn.
Data mining can also help insurance companies identify potential fraud cases by analysing patterns in insurance claims forms, inspector notes, and other relevant data. This not only improves customer service by speeding up legitimate claim processes but also reduces financial losses for the company, freeing up more resources to focus on serving customers.
Furthermore, data mining enables insurance companies to identify new market audiences and sync their strategies with market demand. This helps them attract new customers while also retaining existing ones, thereby reducing customer churn.
Finally, data mining can be used to improve the efficiency of customer support processes. For example, natural language processing (NLP) can be used to automatically route customer support requests to the right departments, reducing response times and improving the overall customer service experience, which can lead to reduced churn.
Understanding CDW Auto Insurance Coverage: Are You Protected?
You may want to see also
Data mining can be used to streamline the process of obtaining insurance quotes
Data mining is an increasingly important process for insurance companies, as they collect a vast amount of data on their customers and claims. Data mining can be used to streamline the process of obtaining insurance quotes in several ways.
Firstly, data mining can help insurance companies understand customer needs and behaviours. By analysing large amounts of data, insurance companies can gain insights into customer motivations, buying patterns, and demographics. This allows them to tailor their products and services to meet the specific needs of their customers. For example, data mining can be used to identify customers who are more likely to accept a price increase, allowing insurance companies to target these customers with specific quotes and policies.
Secondly, data mining enables insurance companies to improve their decision-making process. By analysing historical data and using predictive modelling, insurance companies can more accurately assess risk and set premiums. This allows them to provide customers with more accurate quotes that are based on their individual risk profiles. For instance, data mining can be used to predict a higher auto insurance premium for a customer with a history of rough driving compared to a customer with a lower-risk profile.
Thirdly, data mining helps insurance companies streamline the quoting process by automating and centralizing information. Through the use of advanced analytics and machine learning, insurance companies can quickly process large amounts of data from various sources, such as forms, emails, social media, and claims forms. This allows them to efficiently retrieve and analyse customer information, resulting in faster and more accurate quotes.
Additionally, data mining can assist in fraud detection and prevention. By analysing data trends and identifying red flags, insurance companies can identify potential cases of fraud and mitigate losses. This ensures that legitimate claims are processed promptly, further streamlining the quoting and claims process.
Lastly, data mining enables insurance companies to improve their marketing strategies. By analysing customer data, insurance companies can identify sales opportunities and develop targeted marketing campaigns. This allows them to reach the right customers with the right messages, increasing the likelihood of conversion and improving the efficiency of the quoting process.
In conclusion, data mining offers insurance companies a powerful tool to streamline the process of obtaining insurance quotes. By leveraging data analytics, insurance companies can improve their understanding of customers, enhance decision-making, automate processes, detect fraud, and optimise marketing strategies. These benefits ultimately lead to improved customer satisfaction and business growth for insurance companies.
Southern vs Auto Owners: Insurance Differences
You may want to see also
Frequently asked questions
Auto insurance companies use data mining to design risk-based insurance premiums. They collect a large amount of data on customers and their peers, including demographics, lifestyle characteristics, and risk information. This data is then used to predict the likelihood and expected value of future claims for each insured individual.
Data mining allows auto insurance companies to identify and group high-risk and low-risk customers. This information is then used to determine insurance rates, with high-risk customers paying higher premiums than low-risk customers.
By using data mining, insurance companies can set rates based on a customer's likelihood of filing a claim and the expected value of that claim. This means that customers who are more likely to file claims or have higher claim values will be charged higher premiums.
Auto insurance companies collect a wide range of data, including policyholder attributes (name, address, young driver flag, etc.), car characteristics (make, model year, safety devices, etc.), policy attributes (start and end dates, premium, extras, etc.), and claims details.
Yes, there are ethical concerns and criticisms regarding the use of data mining in auto insurance. Some critics argue that data mining can lead to unfair and discriminatory pricing, especially for low-income customers or those with limited market choices. There are also concerns about privacy and the potential for data misuse.