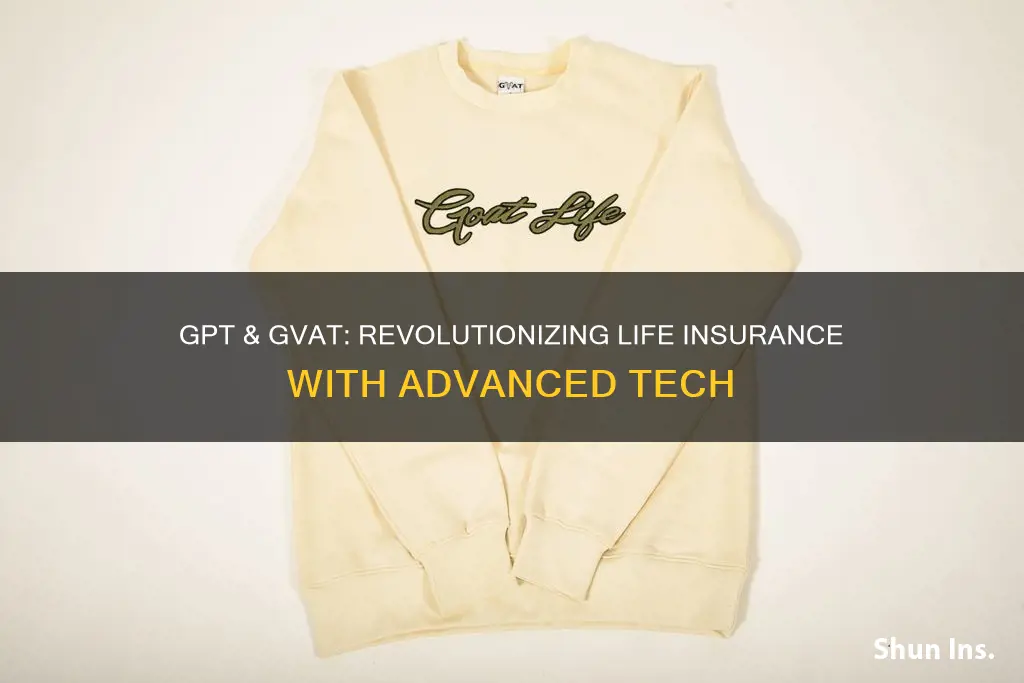
GPT and GVAT are terms that have emerged in the context of life insurance, particularly in the realm of automated underwriting and risk assessment. GPT, or Generative Pre-trained Transformer, is a type of artificial intelligence model that can generate human-like text, which has been utilized in various applications, including insurance. GVAT, or Generative Variable Autoencoder, is a more specialized AI technique that focuses on generating synthetic data to enhance risk modeling and prediction. In life insurance, these technologies are being explored to streamline the underwriting process, improve risk assessment, and potentially offer more personalized and efficient services to policyholders. This introduction sets the stage for a discussion on how these advanced AI techniques are being applied in the insurance industry.
What You'll Learn
- GPT: Generative Pre-trained Transformer, a language model for insurance policy generation
- GVAT: Generative Variational Autoencoder, for data augmentation and fraud detection in insurance
- GPT's Role: Automating policy creation, reducing human error, and improving efficiency
- GVAT's Impact: Enhancing risk assessment, fraud detection, and customer experience in insurance
- Ethical Considerations: Ensuring fairness, transparency, and privacy in AI-driven insurance processes
GPT: Generative Pre-trained Transformer, a language model for insurance policy generation
The Generative Pre-trained Transformer (GPT) is a groundbreaking language model that has revolutionized the field of natural language processing (NLP). It is designed to generate human-like text by predicting the next word in a sequence, given the previous words. This technology has found numerous applications across various industries, and one of its most intriguing uses is in the generation of insurance policies.
In the context of life insurance, GPT can be a powerful tool to streamline the policy creation process. Insurance companies often deal with complex policy documents that require precise language and adherence to specific regulations. GPT's ability to understand and generate coherent text makes it an ideal candidate for automating this task. By training the model on a vast corpus of insurance-related documents, it can learn the structure, terminology, and legal nuances specific to the industry.
The process begins with feeding the model a large dataset of existing insurance policies, contracts, and related documents. Through supervised learning, GPT learns to predict the next word in a sentence, capturing the context and semantics of the insurance domain. This training enables the model to generate coherent and contextually appropriate text, ensuring that the policies are accurate and compliant. For instance, when generating a life insurance policy, GPT can create personalized documents by incorporating the policyholder's details, coverage amounts, and specific terms while adhering to regulatory guidelines.
One of the key advantages of using GPT for insurance policy generation is its efficiency and speed. Traditional policy creation involves manual drafting, which can be time-consuming and prone to errors. With GPT, the model can rapidly generate multiple policy versions, allowing insurers to quickly adapt to changing market demands or customize policies for individual customers. This automation not only reduces operational costs but also enhances customer satisfaction by providing personalized policies promptly.
Furthermore, GPT's ability to learn from vast amounts of data ensures that the generated policies are up-to-date and reflect the latest industry standards. As new regulations or policy trends emerge, the model can be retrained to incorporate these changes, ensuring that the generated documents remain compliant and relevant. This dynamic nature of GPT makes it a valuable asset for insurance companies, enabling them to stay ahead in a rapidly evolving market.
In summary, GPT's application in life insurance policy generation offers a unique blend of automation, accuracy, and compliance. Its language generation capabilities, coupled with industry-specific training, enable insurers to create personalized and legally sound policies efficiently. As the technology continues to advance, we can expect further enhancements in the quality and customization of insurance documents, ultimately benefiting both insurance providers and policyholders.
Life Insurance and THC: Can It Cause Denial?
You may want to see also
GVAT: Generative Variational Autoencoder, for data augmentation and fraud detection in insurance
The Generative Variational Autoencoder (GVAT) is an innovative technique that leverages the power of generative models to address critical challenges in the insurance industry, particularly in data augmentation and fraud detection. This method is a type of generative model that combines the strengths of variational autoencoders (VAEs) and generative adversarial networks (GANs), offering a unique approach to handling complex data patterns.
In the context of insurance, GVAT can be a game-changer. Insurance companies often deal with vast amounts of data, including customer information, policy details, and historical claims. The traditional methods of data analysis and fraud detection may not always be sufficient to identify intricate patterns and anomalies. Here's how GVAT can be applied:
Data Augmentation: Insurance fraud often involves manipulating or fabricating data to claim false benefits. GVAT can be trained to generate synthetic data that closely resembles the real, historical data. By creating diverse and realistic synthetic records, GVAT can augment the training dataset. This augmented data can then be used to train machine learning models for fraud detection, improving their accuracy and ability to identify suspicious patterns. For instance, if a model is trained on synthetic data that mimics fraudulent claims, it becomes more adept at recognizing similar fraudulent activities in the future.
The autoencoder component of GVAT plays a crucial role here. It learns to reconstruct the input data, and by doing so, it captures the underlying structure and distribution of the data. The variational aspect ensures that the generated data is diverse and covers the entire distribution, making it an effective tool for data augmentation.
Fraud Detection: GVAT can also be utilized for fraud detection by learning the latent space representation of legitimate and fraudulent data. The model is trained to generate latent variables that represent the data's characteristics. When new claims or data points are encountered, the GVAT can encode them into the latent space and then decode them to predict the likelihood of fraud. If the decoded output deviates significantly from the expected values, it may indicate a potential fraud case. This approach can help insurance companies flag suspicious activities and make more informed decisions.
Furthermore, GVAT can be integrated with other machine learning algorithms to enhance fraud detection systems. By combining the generative capabilities of GVAT with traditional classification models, insurance companies can create a robust fraud detection pipeline. The generated data can be used to train and test these models, ensuring they are well-prepared to handle various fraud scenarios.
In summary, GVAT offers a powerful solution for insurance companies to tackle data augmentation and fraud detection challenges. Its ability to generate synthetic data and learn latent representations makes it a valuable tool in the insurance industry's arsenal for combating fraud and improving overall data-driven decision-making. As the insurance sector continues to embrace digital transformation, such innovative techniques will play a pivotal role in ensuring a secure and efficient environment.
Life Insurance Trust: Protecting Your Family's Future
You may want to see also
GPT's Role: Automating policy creation, reducing human error, and improving efficiency
The integration of GPT (Generative Pre-trained Transformer) technology into the life insurance industry has the potential to revolutionize policy creation and management. GPT, a type of large language model, can automate various tasks, leading to significant improvements in efficiency and accuracy. One of its primary roles is to streamline the policy creation process, which is often a complex and time-consuming task for insurance professionals.
In the traditional process, creating a life insurance policy involves numerous steps, including data collection, policy customization, and documentation. GPT can automate this entire process by generating personalized policies based on the provided customer information. For instance, when an insurance agent inputs a client's details, such as age, health status, and desired coverage amount, GPT can instantly generate a tailored policy document. This automation reduces the time spent on policy creation, allowing agents to focus on other critical tasks and providing a faster service to customers.
Moreover, GPT's ability to understand and generate human-like text can significantly reduce human error. The insurance industry is highly regulated, and any mistake in policy creation can have serious legal and financial implications. GPT's advanced language processing capabilities ensure that policies are generated accurately and in compliance with relevant regulations. It can verify and validate the input data, ensuring that the policy terms are correct and up-to-date, thus minimizing the risk of errors that might occur with manual data entry.
The efficiency gains from using GPT are also notable. By automating policy creation, insurance companies can process a higher volume of applications in a shorter time frame. This increased productivity can lead to improved customer satisfaction, as faster service means a better experience for clients. Additionally, GPT can assist in identifying and addressing potential issues in policies, further enhancing the overall quality of the product.
In summary, GPT's role in automating policy creation, reducing human error, and improving efficiency is transformative for the life insurance industry. It streamlines processes, enhances accuracy, and increases productivity, ultimately benefiting both insurance providers and their customers. As GPT technology continues to evolve, its impact on the insurance sector is likely to become even more significant, shaping the future of policy management.
Life Insurance Agents: Tech-Proof and Fighting Back
You may want to see also
GVAT's Impact: Enhancing risk assessment, fraud detection, and customer experience in insurance
The integration of Generative Virtual Assistants (GVATs) in the insurance industry is revolutionizing various aspects of the business, particularly in risk assessment, fraud detection, and customer experience. These advanced AI-powered tools are designed to mimic human interactions, providing a more personalized and efficient approach to insurance services.
Risk Assessment: GVATs play a pivotal role in enhancing risk assessment processes. By analyzing vast amounts of data, including customer profiles, medical records, and lifestyle factors, these assistants can identify patterns and trends that may not be immediately apparent to human analysts. This capability enables insurers to make more informed decisions regarding premium pricing, policy coverage, and risk mitigation strategies. For instance, a GVAT can process historical data to predict the likelihood of certain health conditions or lifestyle choices, allowing insurers to offer tailored policies and potentially reduce the overall risk pool.
Fraud Detection: The ability of GVATs to detect and prevent fraud is another significant advantage. These virtual assistants can continuously monitor transactions, claims, and customer interactions, flagging any suspicious activities in real time. By employing natural language processing and machine learning algorithms, GVATs can identify anomalies, such as fraudulent claims, identity theft, or unauthorized access to accounts. This automated fraud detection system not only saves time and resources but also reduces the financial impact of fraud on insurance companies and their customers.
Customer Experience: In the realm of customer experience, GVATs are transforming how insurers interact with their clients. These assistants can provide 24/7 support, answering queries, assisting with policy management, and offering personalized recommendations. By leveraging customer data, GVATs can deliver tailored advice, ensuring that policyholders receive the most relevant and beneficial information. For example, a GVAT might suggest policy upgrades or discounts based on a customer's changing circumstances, thereby improving customer satisfaction and loyalty. Moreover, the instant and accurate information provided by GVATs can empower customers to make more informed decisions about their insurance needs.
The impact of GVATs in insurance is far-reaching, offering a more efficient, secure, and customer-centric approach. These virtual assistants are continuously learning and evolving, ensuring that insurance providers stay ahead of the curve in a rapidly changing market. As technology advances, the potential for GVATs to further enhance risk management, fraud prevention, and customer satisfaction in the insurance industry is immense.
Life Insurance and Mass Health: Incompatible or Not?
You may want to see also
Ethical Considerations: Ensuring fairness, transparency, and privacy in AI-driven insurance processes
The integration of artificial intelligence (AI) in the insurance industry, particularly in life insurance, has opened up new avenues for efficiency and personalization. However, it also raises important ethical considerations that need to be carefully navigated to ensure the well-being of both the insurance providers and the policyholders. One of the primary ethical concerns in AI-driven insurance processes is fairness. Insurance companies must ensure that their AI systems do not perpetuate or exacerbate existing biases. For instance, if an AI model is trained on historical data that reflects societal biases, it might inadvertently deny coverage or offer less favorable terms to certain demographic groups. To address this, insurers should regularly audit their algorithms to identify and mitigate any discriminatory patterns. Additionally, transparent communication about the criteria used for risk assessment and policy pricing is essential to maintain fairness and trust.
Transparency is another critical aspect of ethical AI implementation. Insurers should provide clear explanations of how AI algorithms make decisions, especially in complex processes like underwriting. Policyholders have a right to understand the factors considered in determining their insurance premiums or coverage eligibility. By offering transparent insights, insurance companies can build trust and ensure that policyholders are not left in the dark about the reasoning behind their insurance policies. This transparency can also help in identifying and rectifying any potential errors or unfair practices.
Privacy is a fundamental right that must be protected in the context of AI-driven insurance. As AI systems process vast amounts of personal data, there is a risk of privacy breaches or misuse of sensitive information. Insurance companies should implement robust data protection measures, including encryption, access controls, and regular security audits. Additionally, obtaining explicit consent from policyholders for data collection and usage is essential. Providing users with control over their data, such as the ability to opt-out or request data deletion, empowers individuals and ensures their privacy rights are respected.
Furthermore, the ethical use of AI in life insurance extends to the handling of customer data and interactions. AI chatbots and virtual assistants, for example, should be designed to provide accurate and helpful information while respecting user privacy. These systems should be programmed to handle customer inquiries with sensitivity, especially when dealing with personal and potentially sensitive topics. Regular monitoring and feedback mechanisms can help identify and rectify any privacy-related issues promptly.
In summary, as AI continues to shape the insurance industry, ethical considerations are paramount. Ensuring fairness, transparency, and privacy in AI-driven insurance processes is essential to maintain trust, protect policyholders' rights, and foster a sustainable and responsible business environment. Insurance companies must stay vigilant, adapt their practices, and continuously evaluate their AI systems to meet the highest ethical standards.
Uncover Credit Life Insurance: Check Your Policy Status
You may want to see also
Frequently asked questions
Gross Premium Term, or GPT, is a term used in life insurance to describe the total amount of premium paid by the policyholder over the entire term of the policy. It represents the total cost of the insurance coverage for the specified period. GPT is calculated based on the policy's face value, term length, and the insurance company's rates. This term is crucial in understanding the overall cost and value of a life insurance policy.
Gross Value at Term, or GVAT, refers to the total value of the life insurance policy at the end of its term. It is the sum of all the premiums paid by the policyholder plus any additional benefits or riders that have been added to the policy. GVAT is an essential metric for assessing the overall growth and value of a life insurance investment over time. It takes into account the policy's performance, including any interest or investment returns earned.
GPT and GVAT are key financial indicators that highlight the value and cost structure of life insurance policies. GPT represents the ongoing premium payments, ensuring consistent coverage throughout the term. GVAT, on the other hand, showcases the potential growth and accumulation of value within the policy. Understanding these terms helps individuals make informed decisions about life insurance, ensuring they receive adequate coverage and potentially build a valuable financial asset over time.