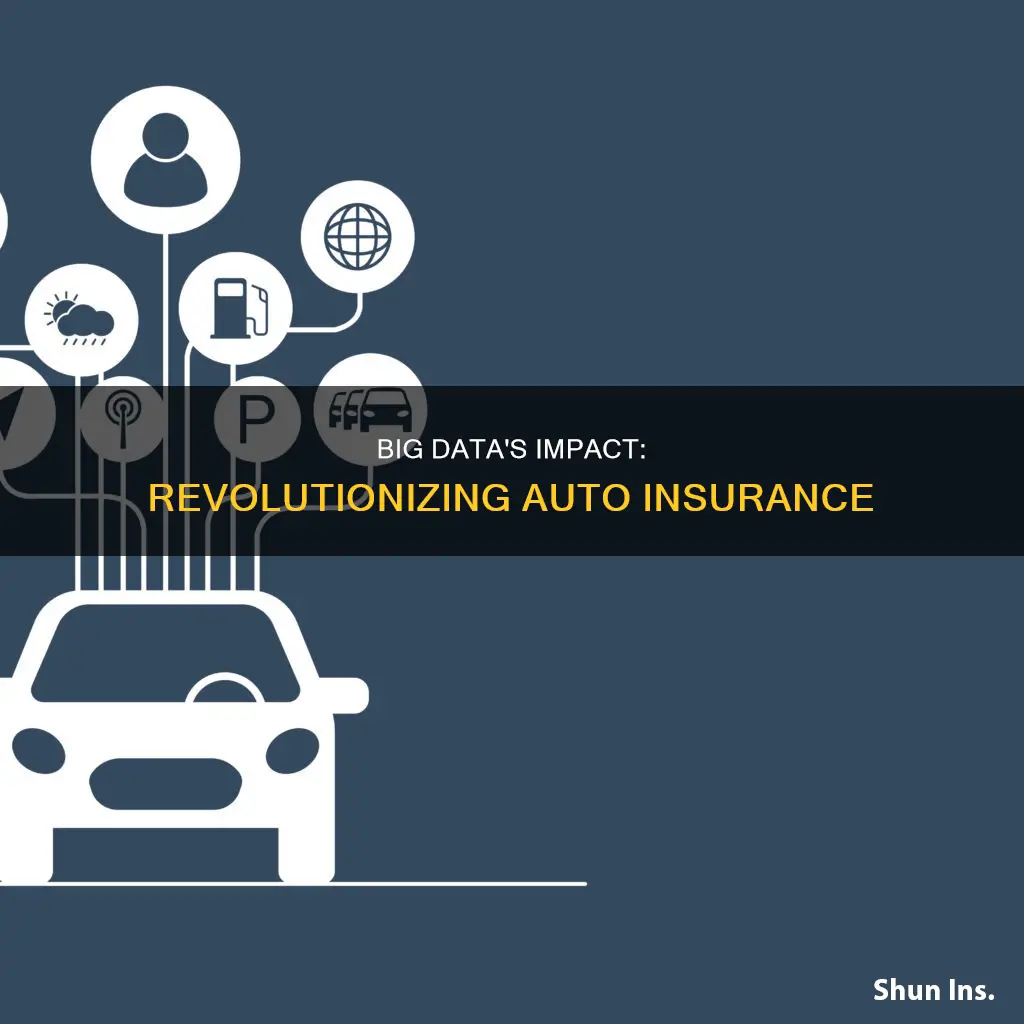
The auto insurance industry is being transformed by big data, which is revolutionizing traditional practices and enhancing customer experiences. Big data provides a vast array of information, from demographics and socio-economic indicators to IoT sensor data, that insurers can use to more accurately understand risk and personalize policies. This data is a goldmine for insurers, enabling them to develop more precise predictive models and improve their understanding of customer needs and preferences. As a result, they can create tailored insurance packages, such as usage-based insurance (UBI) models, and provide responsive customer support through AI-powered chatbots. Additionally, big data plays a pivotal role in fraud detection and enhances the efficiency of claims processing, further improving customer satisfaction. Embracing big data is essential for insurers to stay competitive and meet the evolving needs of digitally empowered consumers.
Characteristics | Values |
---|---|
Data sources | Social media, customer transactions, IoT devices, historical claims, underwriting data, open-source protocols, connected devices, cloud servers |
Data processing applications | Hadoop, Spark, NoSQL databases |
Benefits | Improved risk assessment, personalized policies, enhanced customer experience, fraud detection, streamlined operations, reduced costs, improved profitability |
Drawbacks | Data privacy concerns, ethical use of customer data, cybersecurity threats |
What You'll Learn
Data-driven insights for risk assessment and policy customisation
The integration of big data in the auto insurance industry has brought about a shift from traditional methods of risk assessment to data-driven insights, enabling insurers to offer customised policies to their customers.
In the past, insurance companies relied on manual, traditional methods of assessing risk, which often involved collecting and analysing data from limited sources, such as historical claims and underwriting data. This often led to inaccurate risk assessments and incorrect pricing, resulting in significant losses.
Big data, on the other hand, provides a vast array of information from diverse sources, including social media, customer transactions, IoT devices, and government statistics. By leveraging the power of big data analytics, insurers can now make more informed decisions about risk assessment. They can analyse historical data to predict the likelihood of future risks and take proactive measures to mitigate them. For example, by analysing data from IoT sensors, insurers can gain insights into driving behaviour, vehicle performance, and journey details, which can be used to create tailored insurance packages that reward safe driving habits with lower premiums. This not only benefits the insurer by reducing potential losses but also the insured, who can enjoy lower insurance costs.
In addition to improving risk assessment, big data also enables insurers to customise their policies to meet the specific needs and preferences of their customers. By analysing customer data and behaviour, insurers can develop targeted marketing campaigns and create personalised insurance offerings. For instance, usage-based insurance (UBI) models in the auto insurance industry use telematics data to price policies based on individual driving habits such as speed, braking patterns, and mileage. This approach not only benefits insurers by allowing them to price their products more accurately but also enhances customer satisfaction by providing policies that better match their specific lifestyles and risk profiles.
The use of big data in risk assessment and policy customisation within the auto insurance industry is a powerful example of how data-driven insights can revolutionise traditional practices, improve accuracy, and enhance the overall customer experience.
ACV or Replacement Cost: Understanding Mercury Auto Insurance's Payout Options
You may want to see also
Streamlined operations and enhanced customer experiences
The integration of big data, machine learning, and AI technologies is revolutionizing the auto insurance industry by streamlining operations and enhancing customer experiences.
Big data analytics enables auto insurance providers to analyze vast amounts of data from various sources, including social media, customer transactions, and IoT devices. This data analysis helps insurers identify potential customers most likely to purchase their products by understanding their behavior, preferences, and buying patterns. As a result, insurers can develop targeted marketing campaigns, optimize their marketing efforts, save time, and increase sales.
For instance, AIG offers an Internet-enabled app called 'XLNT Driver', which records driving performance and scores journeys. This data is then shared with AIG, allowing them to create tailored insurance packages, ultimately offering better insurance packages and encouraging safer driving. Similarly, AXA offers the 'DriveSafe' app for drivers under 24, which records journeys and shares data with the insurer. Using these scores, AXA can offer insurance discounts to drivers.
Big data also plays a pivotal role in streamlining claims processing and enhancing customer satisfaction. Insurers employ algorithms to automate claims intake, extract relevant information from documents, and expedite claims handling, reducing processing times and improving efficiency. Additionally, big data enables insurers to leverage data to assess car damage, further expediting claims processing and increasing customer satisfaction.
Furthermore, big data facilitates a deeper understanding of customer needs and preferences, enabling insurers to provide personalized services and interactions. Chatbots, AI-powered customer service, and data-driven insights empower insurers to deliver proactive and responsive customer support. For example, Abacai's boom, a digital car insurance service, offers an AI-powered chatbot available on its mobile app and web portal. The chatbot provides 24/7 support and assists policyholders with queries related to policies and the claims journey, resulting in an enhanced customer experience.
The utilization of big data in the auto insurance industry has transformed operations and improved customer experiences. By analyzing large datasets, insurers can develop targeted marketing campaigns, streamline claims processing, and provide personalized services, ultimately increasing customer satisfaction and retention.
Auto Insurance Comprehensive Deductibles Explained
You may want to see also
Fraud detection and financial loss prevention
The insurance industry has long been susceptible to fraud, with fraud detection a challenging problem given the variety of fraud patterns and the relatively small ratio of known frauds in typical samples. Fraudulent activities range from staging incidents to misrepresenting situations, actors, causes, and the extent of damage.
Big data analytics can be used to detect insurance fraud by identifying abnormal claims and creating new sets of tests to automatically narrow the segment of potentially fraudulent applications or detect new patterns of fraud. This can be achieved by applying business rules, anomaly detection, text mining, database searches, and social network analysis.
For instance, in the auto insurance industry, telematics devices can be used to transmit data in real-time, which can be analysed to develop more accurate pricing, improve risk management techniques, and reduce losses by enabling better claims assessments. This data can include information such as date, time, speed, longitude, latitude, acceleration or deceleration, cumulative mileage, and fuel consumption.
Big data can also be used to train tools to calculate claims following accidents and reduce future healthcare costs. In the health insurance sector, data mining tools and techniques can be used to detect fraud in large sets of insurance claim data. By analysing past insurance claims, the anomaly detection technique can calculate the likelihood of a record being fraudulent.
In addition, machine learning techniques can be used to improve predictive accuracy, enabling loss control units to achieve higher coverage with low false-positive rates. Techniques such as feature engineering, feature selection, and parameter tweaking can be explored to achieve superior predictive performance.
Who Pays for the At-Fault Driver's Medical Bills?
You may want to see also
Improved marketing and sales through targeted campaigns
The auto insurance industry is undergoing a significant transformation due to the integration of big data, with companies utilising it to improve their marketing and sales strategies. By collecting and analysing vast amounts of data from various sources, such as social media, customer transactions, and IoT devices, insurers can develop targeted marketing campaigns that are more likely to convert leads into customers.
One way big data is revolutionising marketing and sales in the auto insurance industry is through telematics. Telematics refers to the use of wireless devices to transmit data in real time to insurance companies. This data includes information such as date, time, speed, longitude, latitude, acceleration, deceleration, cumulative mileage, and fuel consumption. By analysing this data, insurers can develop more accurate pricing models, improve risk management techniques, and reduce losses by enabling better claims assessments. For example, insurers can offer pay-as-you-drive (PAYD) or pay-how-you-drive (PHYD) plans, where premiums are based on the distance driven and driving habits such as speed, cornering, and harsh braking.
In addition to telematics, insurers are also using driver behavioural analytics to create tailored insurance packages. For instance, AIG offers an app called 'XLNT Driver' that records driving performance and shares this data with AIG, allowing them to create customised insurance packages. Similarly, AXA offers the 'DriveSafe' app for drivers under 24, which records journey data and provides insurance discounts for safe driving. These apps work on the principle of sharing data for something back, creating value for the consumer.
Big data also enables insurers to identify potential customers who are most likely to purchase their products. By analysing customer behaviour, preferences, and buying patterns, insurers can develop targeted marketing campaigns that resonate with their target audience. This helps insurance companies optimise their marketing efforts, save time, and increase sales.
Furthermore, big data allows insurers to provide personalised solutions and services to their customers. By gathering and analysing large amounts of customer data from social media, feedback, and transactional data, insurers can gain insights into customer preferences, needs, and behaviour patterns. This enables them to offer tailored products and services that meet the specific needs and preferences of their customers, improving customer satisfaction and loyalty.
In conclusion, big data is transforming the auto insurance industry by enabling improved marketing and sales through targeted campaigns. Insurers can now utilise data to develop customised insurance packages, identify potential customers, and provide personalised solutions, resulting in increased sales and improved customer retention.
Does Savemore Auto Insurance Cover Rebuilt Titles?
You may want to see also
Dynamic pricing and increased profitability
Dynamic pricing is a practice popularized by Uber, which enables insurers to respond and act upon real-time market changes, aligning with consumers' expectations. Dynamic pricing allows insurers to deploy prices that reflect consumers' expectations, offering cheaper policies to low-risk customers and a different premium model for high-risk policyholders. This involves assessing how much risk a customer represents by factoring in usage and risk-averse behaviors, and allowing pricing to evolve as circumstances change.
Traditionally, gathering data to deploy rates can take several months, due to the nature of legacy systems. However, dynamic pricing enables insurers to respond to changes in consumer preferences and behaviors in real time, ensuring compliance and governance over the rate-making process. This allows insurers to realize value almost immediately, as they can adapt to changing customer circumstances and offer timely rates.
Dynamic usage-based insurance (UBI) models, for example, can capture and analyze vast amounts of telematics data, allowing insurers to transition from standardized purchase and annual renewal models to a continuous cycle where offerings are constantly adapted to individuals' behavioral patterns. This also enables the growth of the sharing economy, offering pay-by-mile for car-sharing services or pay-by-stay for home-sharing services.
The use of telematics data, collected by onboard 'black boxes', is a significant factor in dynamic pricing for auto insurance. Telematics data can include mileage, date, time, location, speed, cornering, harsh braking, and frequent lane changes. This data enables insurers to develop more accurate pricing models, improve the granularity of risk management techniques, and reduce losses by enabling better claims assessments.
The adoption of dynamic pricing in the insurance industry is a response to the digital transformation and the increasing consumer demand for convenience and personalized policies. By investing in innovative technologies, insurers can improve operational agility, drive real value, and cater to evolving consumer demands.
Insurance Gaps: Hidden Costs Revealed
You may want to see also
Frequently asked questions
Big data refers to the massive volume of structured and unstructured data collected from various sources, including social media, customer transactions, and IoT devices.
Big data helps the auto insurance industry by providing a more accurate understanding of their customers and their risks. This enables insurers to offer personalized products and services, improve customer satisfaction and retention, and make more informed decisions about pricing and risk management.
By analyzing large amounts of data from various sources, insurers can identify emerging risks and develop strategies to address them. This helps them to price their products appropriately, reduce claims, and improve profitability.
Big data analytics can identify patterns of fraudulent activities and detect anomalies in claims data, enabling insurers to investigate suspicious claims and prevent fraud. Machine learning algorithms continuously monitor data and identify new types of fraud, helping insurers minimize losses and maintain financial stability.